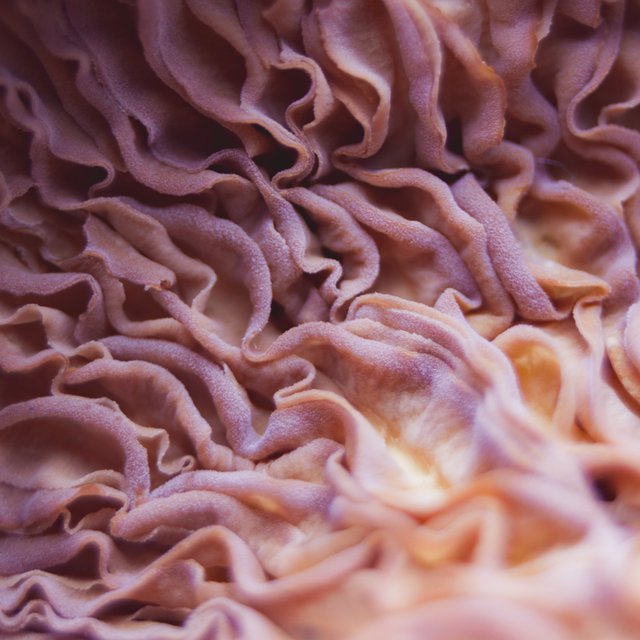
NEUROTECH educational seminar #2: What are hardware opportunities for neuromorphic engineering?
A review
1 February, 2021, by Michael Schmuker
Each month, NEUROTECH holds an educational webinar where experts from the field explain the fundamentals of neuromorphic technology. The second instalment of the NEUROTECH educational webinar ran on 1st December 2020. In this particularly lively episode, we had four experts speak about various approaches towards neuromorphic hardware.
Johannes Schemmel (KIP Heidelberg) spoke about scaling up neuromorphic technology in size and speed. Johannes described how today’s machine learning still is still based on biological knowledge that was prevailing 70 years ago. The hope is to solve artificial intelligence problems more efficiently by deepening our understanding of biological learning and, at the same time, building new hardware that closely mimics key principles found in the brain. But neuromorphic systems need to scale up tremendously in size, speed, complexity and learning capabilities to compete with the current state of the art in machine learning, while maintaining energy consumption on a reasonable level. Johannes described the various hardware-“tricks” that his group employed in the design of the BrainScaleS neuromorphic system to achieve its unmatched combination of speed, complexity, and flexibility in learning capabilities.
Jennifer Hasler (Georgia Tech) gave her historical perspective on floating gate devices and how they shaped today’s neuromorphic computing. Floating gate devices are circuit elements that can store and read out a programmable charge and therefore can act as memory, e.g. in synaptic circuits. Jennifer gave an overview on the electronic fundamentals and wizardry involved in synaptic memory, to the autozeroing floating-gate amplifier, and large-scale Field-Programmable Analog Arrays (FPAAs), the mixed-signal homologue to FPGAs. We also got some juicy insight into DARPA's Sinapse programme and the role of Floating gates vs. digital technology in the development that ultimately led to TrueNorth. I won’t give away the details here but instead recommended you watch the recording of Jennifer’s entertaining talk!
Elisa Vianello (CEA LETI) started by the point that AI needs new hardware to cope with rising energy demands of model training. Since data movement incurs by far the largest share of power consumption, on-chip memory and resistive switching memory will play a crucial the crucial in the future development of AI hardware. Various memristor implementations exist, such as such as phase-change, filamentary switching, magnetic memories, and they are already integrated into foundry processes. Elisa presented an embedded example scenario and two approaches for scaling towards on-chip high density memory. A particular challenge that will have to be overcome is posed by sneak currents, that occur when reading out resistive memory in a passive crossbar design. The potential of resistive memory lies in near- and in-memory computing. As an example, Elisa showed how resistive memory can be incorporated in with electronic neurons in spiking network neuromorphic devices, where programmable resistive memory could provide synaptic plasticity for on-chip learning.
Bert Offrein (IBM Research) talked about Analog signal processing for Neuromorphic Computing. He started with a quick tour of ANNs and backpropagation. Multiply-accumulate (MAC) operations dominate the computational load in these applications. Bert highlighted the advantages of analogue crossbar arrays in this context, that allow MAC operations in one timestep, independent of the number of MAC factors. This is a huge advantage over conventional systems, that require separate operations for each factor. Crossbar arrays therefore combine parallel and in-memory computing with analogue signal processing. But analogue signal processing to implement proper MAC operations, memristive devices must support smooth control of their resistance, something that many current technologies are not capable of. Bert showed that smooth resistance control in memristive devices can be achieved by incorporating a metal oxide sheet as a switching layer. Their new device allows to address intermediate resistance states and therefore enables power-efficient analogue MAC operations in a crossbar array.