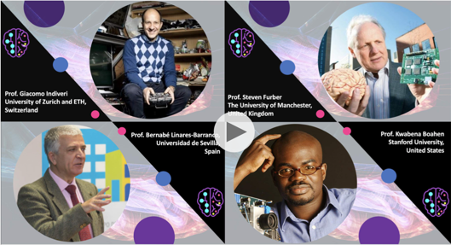
A review of the first NEUROTECH educational webinar
23 January, 2021, by Michael Schmuker
Part of NEUROTECH’s mission is to build and provide educational resources that harness and disseminate the knowledge on Neuromorphic Technologies that is available in the research community. To this end, we have launched a series of monthly seminars, where experts from the neuromorphic research community speak on fundamental research concepts as well as cutting-edge findings from their labs. Seminars are recorded and published on our NEUROTECH Educational Video Channel.
The first seminar was held on Nov 3, 2020. Four speakers spoke on the topic “What is Neuromorphic Computing?”
Giacomo Indiveri (ETH Zürich) spoke about the history of neuromorphic computing and how it started with Carver Mead and Misha Mahowald. He covered how Neuromorphic computing evolved from pure analog circuits modeling neurons and networks to today’s plethora of technologies, including mixed-signal and purely digital systems, in-memory computing and memristive devices. Giacomo covered in detail the subthreshold analog circuits that model the electronic processes that provide the computational properties of biological neurons, and their integration in neuromorphic processors.
Steve Furber (University of Manchester) explained how he became involved in neuromorphic computing. Steve started out designing custom chips for the BBC Microcomputer (1982) and then leading the group that developed the first ARM chip in 1985. He joined the University of Manchester in 1990 and led the design and development of asynchronous ARM processors. But after 20 years of skyrocketing progress in general purpose computing, computers still struggled to do things that humans found easy. This was the question that drove Steve to develop the SpiNNaker project, which harnesses a million ARM cores to support real-time models of brain subfunctions. In his talk, Steve described the innovative neuromorphic aspects of the SpiNNaker system and how its goal is primarily to contribute to Neuroscience, while the technology is also useful for prototyping neurorobotic control systems.
Bernabé Linares-Barranco (Universidad de Sevilla) emphasised that computation with timed events is the basis of the low-latency and low-power information processing, inference, and control capabilities of the brain. Starting from Simon Thorpe’s famous work that demonstrated how humans achieve face detection using a single spike per processing layer, Bernabé described how his group developed ultra-rapid visual object recognition based on pseudo-simultaneous processing in a hierarchical event-based convolutional network. The performance of their system is demonstrated by the very impressive real-time recognition of symbols during flipping through a stack of cards.
Finally, Kwabena Boahen (Stanford University) highlighted how three-dimensional structure of compute units is key to efficient brain-like computing. State-of-the-art AI networks like GPT-3 achieve human-like performance but require a huge number of parameters, in the order of 10^11. What’s more, their performance follows a power-law with regards to the number of parameters used, and therefore also with the amount of memory required to deploy such a network. 3D-integration of memory provides a way to fit the huge amount of required memory into small devices like a phone. Kwabena explained how a neuro-inspired approach to memory access that sparsifies signals based on unary instead of binary encoding may lead to dramatic savings in energy that may enable having state-of-the-art AI networks on power-constrained devices like phones.